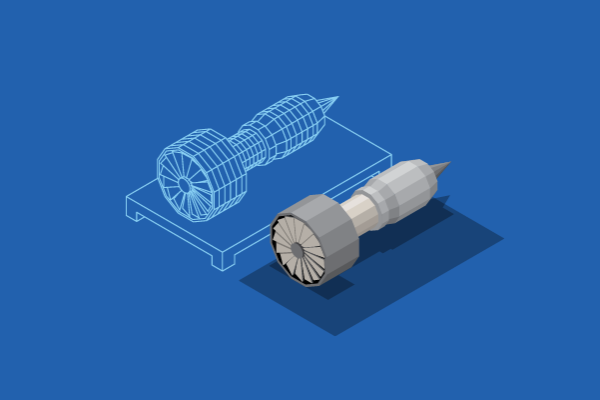
With the Digital Twin, enterprises can gather critical data from assets, for analysis. They can optimize processes, predict maintenance needs, improve products and services, and predict future needs. IoT Interoperability opens up these possibilities that AI and the digital twin promise…
If a car’s brakes were close to failing, wouldn’t it be handy if the car could feel pain, so it slows to a limp, and stops off at the doctor’s to get its brakes fixed. A digital twin, whether it’s a twin of car components, of manufacturing kit, a device in a power plant, or any number of other Things, is plugged into a collection of nerves to gather critical data for analysis, for your action.
We can wire our machines with nerves all day long, but we’re going to be in a tangle if that’s where we stop. We need to know what’s important. The catch on the car trunk is worth knowing about, but less critical than the brakes – obvious in that scenario; but what are the things to measure in your operations? The term ‘digital twin’ can suggest something it’s not – it’s not a clone of every square inch of the machine. Just the critical bits.
There’s much chatter about traditional operations and maintenance processes falling by the wayside as machinery and assets send a stream of data back to base, keeping us informed on operating conditions, so we can take the right action right when it’s needed. But even when we’ve identified the right parts of the system to observe, there’s a lot of data in the stream, and pulling out the key stories from it, so we can keep assets operating smoothly, is not super simple.
A digital twin enables us to predict the impact of different scenarios. Under ‘x’ conditions, with ‘y’ usage, what’ll be the wear and tear? Traditionally, maintenance schedules are based on worst-case scenarios, and so assets get more maintenance than they need, which costs more than it need; and elsewhere weak spots are not spotted until something breaks. Run simulations of many different scenarios across assets to be counted in the thousands in many cases, add in data from similar machines, and action based on AI analysis on the Digital Twin can save organizations considerable time and money.
When you know about the weak spots in machinery as they emerge, you can order spare parts or new assets in advance, schedule maintenance more efficiently, and cut downtime. Weak spots may not only be in machinery – but also in operations – these operations weak spots you might never have discovered without nerves wired into the important points, and AI analyzing what’s going on. Now, engineering, purchasing, HR, whatever is needed to fix the weak spots, can spring into action.
With better informed decisions integral to the organization’s objectives – rather than reactive to mishaps – we’re optimizing production, driving down costs, and likely introducing new operational business models. Data can also feed into design of machinery and assets, if the data is shared with the equipment manufacturer, or the equipment manufacturer has nerves wired in. We also have our hands free to concentrate on the value the project will bring, and we can give the next project more focus. Our days are about making progress more than they are about fighting fires.
We can be sure that the operations of one device will impact the operations of another type of device or another system, and with many devices across our operations, interactions multiply. Even where we as humans can’t see clearly what the impacts at the ends of these threads might be, and how we might make things work better, AI can help us find the tweaks we’ll need to make to uncover value. With an open standards IoT platform built for interoperability, such as plgd, we have threads of data all in the same native language, meaning AI can better weave the threads into a coherent story. The possibilities for increased efficiency and productivity, better informed decision making, new business models, become realities. IoT Interoperability opens up possibilities that AI and the digital twin promise.
plgd makes it simpler to build a successful IoT initiative – to create a proof of concept, evaluate, optimize, and scale.