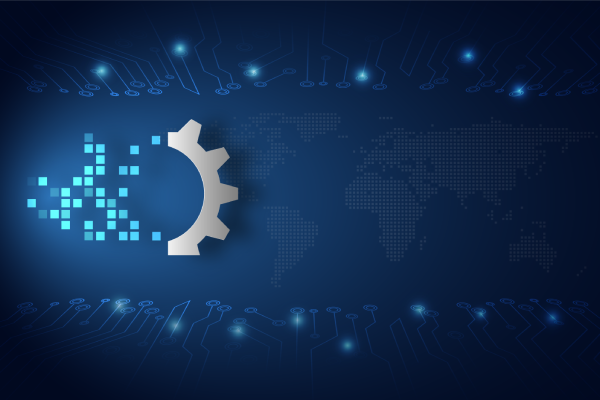
The gulf between Smart Factories and the less smart is deepening. Manufacturers ahead in the game are seeing competitive edges through evolutions of the human-robot team, predictive maintenance, optimizing output, AI in design, and supply chain, all supported by Industry 4.0 cybersecurity.
The gulf between Smart Factories and the less smart is deepening. IoT and AI are enabling manufacturers to channel their data into a single brain, which means much better clarity, analysis, and asset utilization. Competitive edges come from human-robot collaboration and empowering human workers, with AI bringing advantages in predictive maintenance, improving output quality, evolving the design process, and enabling manufacturers to get a better handle on their supply chains.
Calvo and Gil state that increases in productivity up to 50% are possible with collaborative robots. I think we can add to this that human-robot collaboration is a book waiting to be written. The possibilities are endless.
Robots do heavy work and highly ergonomic work well. While humans do problem solving and flexibility better, even where AI aids them in those tasks. So you need your people. This isn’t going to change in the short and medium terms.
The advantage to find here is in the human-machine interface – how can your humans and your robots add to each other?
Once you find these solutions, workers need to know that your focus is for machines to assist them as you assess how to grow value, and enable them in the new production environment. Otherwise the evolution won’t work – because this digital transformation is a process of figuring out ‘how’ – not a simple switchover.
Re-skilling current workers is good for them, and good for you, because you’ll need buy-in from the people in the manufacturing plant. 1. To assess how best to transform. 2. To have people implement the changes over time.
This is one of the big value adds. In one example, an automobile manufacturer reduced unplanned downtime of a critical asset by 25 percent. And even where predictive maintenance is not the answer, frequently other digital reliability programs, are. In a predictive maintenance program, regular scheduled maintenance slots are canned; machine learning takes the lead, spotting anomalies, predicting failures of components or systems and sending alerts so the truck can roll. Downtime and costs are reduced, with maintenance only happening when and where it needs to. And machine life is lengthened, with fixes done promptly, so further damage is prevented.
Every instance of waste, low output, or issue in quality, impacts the bottom line and hands the competition an opportunity. But manufacturers have long sought to optimize, and they struggle to optimize their processes much further. Plus, the causes of some inefficiencies are simply not apparent.
AI can help pinpoint the root cause, and find issues we weren’t aware of. Machine learning algorithms learn every aspect of the production process, identifying trends and patterns in data.
With analysis of the data, AI generates recommendations to help production teams boost efficiency or fix quality issues or system issues.
McKinsey reports that across a wide range of sectors it is not uncommon to see 10 to 30 percent increases in throughput. Improvements in throughput, and in quality, and a reduction of scrap are often realized all at once.
AI in the form of generative design applications can help you explore solutions and permutations more rapidly, and produce more progressive designs. To an AI, you might specify constraints such as turnaround and cost, as well as variables such as raw materials and manufacturing methods. The solutions generated can then be tested by a machine learning algorithm against different manufacturing scenarios, and in augmented reality, iterating to optimize the solutions.
AI can help manufacturers anticipate changes in demand and optimize inventory, warehousing, staffing, last-mile delivery, energy, and raw materials, and make better strategic decisions. Algorithms can analyze and predict demand based on many factors, including the socioeconomy, macroeconomy, political events, and weather.
McKinsey has seen drops in forecasting errors of 20% to 50%, translating into reduction of lost sales and product unavailability by 65%, and 5%—10% reduction in warehousing costs.
As more and more devices are connected, the potential for cyber attacks is growing exponentially, and any shutdown in manufacturing, no matter how brief, can be costly. AI is valuable here, more accurately detecting attacker behaviors in real time across cloud, data center, IoT, and enterprise networks, enabling faster responses, to secure production facilities.
Your needs will include sensors to capture live data, data aggregation, connectivity via routing, gateways, and communication protocols, integration with PLCs, AI applications, and dashboards. See how the plgd IoT platform has enabled others and can enable you to get the most out of AI. You can also book a meeting with us to explore the possibilities for your manufacturing facilities.
plgd makes it simpler to build a successful IoT initiative – to create a proof of concept, evaluate, optimize, and scale.